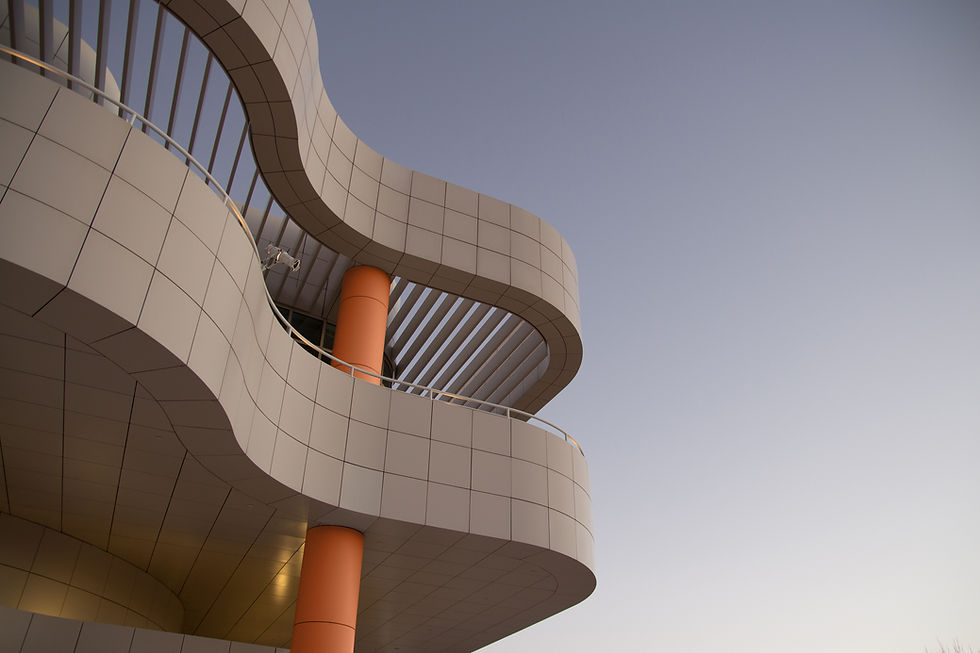
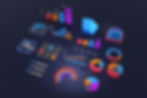
Artificial Intelligence (AI) has rapidly become a transformative force across industries. While generative AI, with its ability to create new content, often grabs headlines, discriminative AI is poised to deliver significant business benefits. Discriminative AI models excel at analyzing existing data to identify patterns, trends, and anomalies. This capability is crucial for tasks like fraud detection, customer segmentation, and predictive maintenance.
The Distinction Between Discriminative and Generative AI
Discriminative AI:Â Focuses on classifying or predicting outcomes based on input data. It learns to distinguish between different categories or make accurate predictions. #DiscriminativeAI #AI #MachineLearning
Generative AI:Â Creates new content, such as text, images, or code, by learning from existing data patterns. It generates novel outputs that resemble the training data. #GenerativeAI #AI #MachineLearning
While generative AI has its applications, discriminative AI offers more immediate and practical benefits for businesses. By analyzing historical and real-time data, discriminative models can:
Identify anomalies:Â Detect fraudulent transactions or equipment failures early on. #AnomalyDetection #FraudDetection #PredictiveMaintenance
Optimize operations:Â Improve efficiency by predicting maintenance needs or optimizing supply chains. #Optimization #SupplyChainManagement
Personalize customer experiences:Â Tailor marketing campaigns and product recommendations based on individual preferences. #CustomerExperience #Personalization
Data Quality: The Foundation of AI Success
To effectively leverage discriminative AI, businesses must prioritize data quality. The accuracy, completeness, and integrity of the data fed into AI models directly impact the quality of their outputs.
Accuracy: Ensure data is free from errors or inconsistencies that could lead to incorrect predictions. #DataQuality #DataAccuracy
Completeness: Gather comprehensive data to capture the full range of relevant variables. #DataQuality #DataCompleteness
Integrity: Maintain data security and privacy to protect sensitive information. #DataQuality #DataSecurity #DataPrivacy
Preparing Your Business for AI Adoption
If you're considering incorporating AI into your operations, a thorough evaluation of your data infrastructure is essential. This involves:
Assessing data quality:Â Identify and address any issues that could hinder AI performance. #DataQualityAssessment #DataCleaning
Standardizing data formats:Â Ensure consistency across different data sources. #DataStandardization #DataIntegration
Implementing data governance:Â Establish policies and procedures to manage data effectively. #DataGovernance #DataManagement
By investing in data quality and governance, businesses can lay a strong foundation for AI adoption and reap the rewards of discriminative AI applications.
Embrace the Power of Data - Today!
By focusing on actionable insights, user-friendly solutions, and outsourced expertise, mid-sized businesses can unlock the hidden potential of their data. Don't get bogged down in complexities - Klout can help you transform your data into a competitive advantage.
Ready to take the first step? Contact Klout today and see how we can simplify your journey to data-driven success!